The world is progressing at a highly rapid pace. The rise of machine learning has disrupted our lives and has changed how we do business. And it is evident that many people have been talking about the use of machine learning (ML) in industries.
What Is Machine Learning?
Machine learning is a subset of artificial
intelligence (AI) in which machines can learn without being explicitly
programmed. This type of AI is based on algorithms and statistical models.
Machine learning can also be used with other technologies to solve problems.
For example, it can be combined with Big Data.
There are many ways machine learning can be
applied in the business world. One such is the application of machine learning
models. Machine learning models employ algorithms and data analysis to predict
future trends and outcomes.
It allows businesses to better prepare for what
is to come and be better informed about their customers. For example, a machine
learning model can predict a customer's future purchases based on the trend of
their previous purchases. This allows businesses to determine the best prices
and products to sell.
It is essential not to forget the model's output
and algorithm when considering machine learning models. Different models and
algorithms are meant to solve other problems. However, when a suitable model is
used, it can be the difference between a solution being created and a problem
remaining unsolved.
Machine learning model operations require the
output and input of data from the user. Thus, the user can determine if the
model is performing as they need it to.
ModelOps is the process of monitoring and analyzing
machine learning models. It helps you keep your models up and running. As a
result, we can significantly reduce the time to perform operations. It can make
the process more resilient, which is vital when working with large volumes of
data.
Once the data is correctly inputted and the
model is oriented correctly, the user can predict the output of a given data
set. It can allow the user to predict future events in various fields and
industries.
Machine Learning Technology in Your Business
Machine learning technology is making the world
a better place. We've all heard about self-driving cars and how they will
change the way we do things. Here are three things you can do with machine
learning technology in your industry:
- You could use it to analyze
data and see if it is worth pursuing or if it is not.
- It can save time by
predicting the success of a product or if a
product will have a lot or little return on investment.
- If you're using much manual labor to do tasks, machine learning can help you automate the process. So, it takes less time to get the same results and increases your overall productivity.

There are two common ways to deploy machine
learning into your business.
1. Batch Inference
Batch inference operates regularly and returns
results for the latest set of new data collected since the last iteration.
Batch inference produces responses with some delay.
Thus, it's beneficial when you don't require
model findings right away. Batch inference can allow you to deploy more sophisticated
models with more high accuracy because there is no delay restriction.
A banking firm can implement a credit assessment
system with a batch inference that operates once a day. Because there is no
requirement to update ratings in real-time. Customers' credit scores could be predicted using the model, which would
be based on the latest data from the previous day.
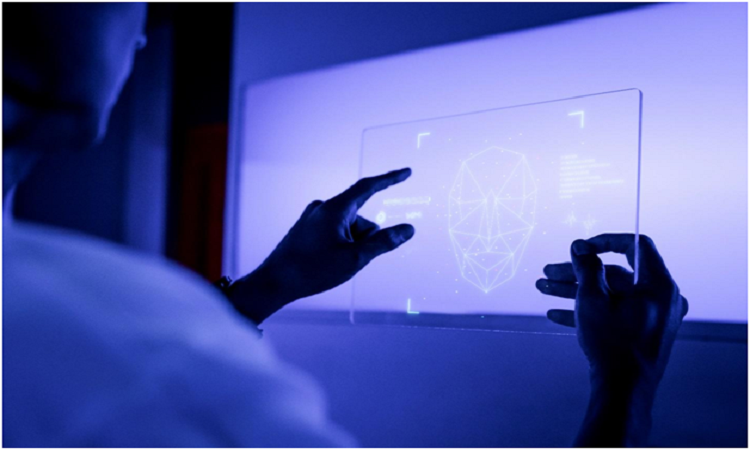
Imagine using an e-commerce site like Amazon to
get personalized suggestions. Data scientists may elect to make suggestions for
users in bulk. It can store them for quick retrieval when required.
2. Online Inference
The system is accessible 24/7 and gives findings
in real-time and on-demand via online inference, also known as real-time
inference. This appears to be a positive attribute in most circumstances. The
delay restriction reduces the kind of ML models you may use.
You can't employ sophisticated models with
online inference because it needs to offer findings in real-time. Furthermore,
the simulation must be capable of executing at any moment. It is more
operationally demanding.
This creates a whole new world of possibilities
for machine learning applications. Instead of waiting hours for forecasts to be
made, we may generate estimates as required and provide them to users
immediately. We can also create predictions for any new data using online
inference.
Furthermore, robust monitoring methods are
required for online inference systems. Data scientists should watch the
distributions of both raw data and the output predictions.
If these distributions aren't the same, it's
possible that an error occurred somewhere in the data flow. It could also
indicate that the data-generating mechanisms have altered. We can make
predictions as soon as necessary and deliver them to users immediately.
For example, an approximate time-to-delivery is
produced when a customer purchases a meal through UberEATS. It would be impossible to create a batch of
these estimations and then distribute them to consumers.
Consider the amount of time required for your
order to come until it has arrived. Any consumer-facing app that allows users
to question models in live time is all forms of applications that can improve
from online inference. Suggestions may also be provided online, based on the usage
situation.
Final Thoughts
Machine learning technology is only as practical
as it is functional for the application you're trying to put it in. Determine
what problem you're trying to solve, and you'll have a good idea of where to
start. Next, you need to identify what data you're going to analyze.
It will help you figure out what is worth your
time. If you have vast amounts of data, you'll want to pick and choose what you
analyze to gain the insights you need.
And finally, you need to test the product. The
best way to test the outcome will depend on the application and the kind of
data you're working with. When in doubt, try out several different options.
When determining how to implement your machine
learning algorithm, one of the first concerns you'll have to address is whether
to employ batch inference or online inference. Product considerations mainly
influence this decision.
Who will be using the assumptions, and when will
they be required? The batch inference is a good option if the forecasts do not
have to be delivered right away. If you need to make unique forecasts when it
takes to make a single online request, the online inference is the method to
go.
Read More>>> https://www.businesstechworld.com/2022/03/protect-your-small-business-from-legal-liability.html